

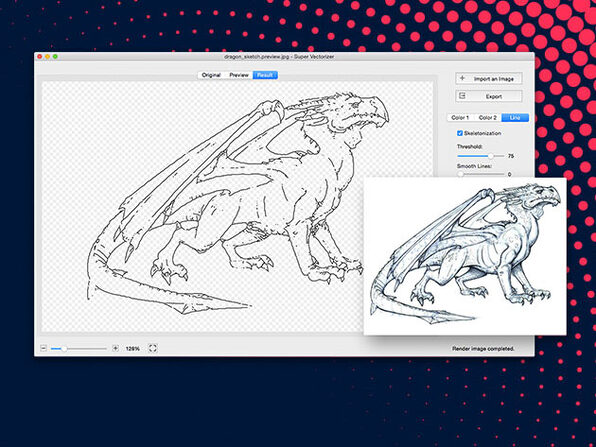
That the sign bit of MurmurHash3 is independent of its other bits. The present implementation works under the assumption To determine the column index and sign of a feature, respectively. Used two separate hash functions \(h\) and \(\xi\) The original formulation of the hashing trick by Weinberger et al. In the following, “city” is a categorical attribute while “temperature” Identifiers, types of objects, tags, names…). To a list of discrete of possibilities without ordering (e.g. Categoricalįeatures are “attribute-value” pairs where the value is restricted Need not be stored) and storing feature names in addition to values.ĭictVectorizer implements what is called one-of-K or “one-hot”Ĭoding for categorical (aka nominal, discrete) features. While not particularly fast to process, Python’s dict has theĪdvantages of being convenient to use, being sparse (absent features NumPy/SciPy representation used by scikit-learn estimators. The class DictVectorizer can be used to convert featureĪrrays represented as lists of standard Python dict objects to the Is a machine learning technique applied on these features. Images, into numerical features usable for machine learning. The former consists in transforming arbitrary data, such as text or Feature extraction is very different from Feature selection:
